The Laws Of Automation, NTT Research Details ‘Physics Of AI’ Group
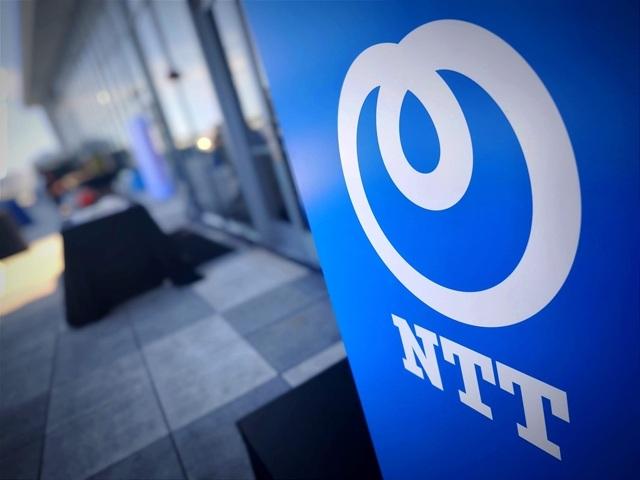
NTT has an expansive platform of software services from an equally broad base of hardware.
AI, like life, is complicated. As the development of artificial intelligence and machine learning technologies has variously spread, skyrocketed and occasionally snowballed, we have sought to understand precisely how our new intelligence engines have gained their capabilities. Not necessarily due to “rise of the robots” style fearmongering centered around any suggestion that the machines will take over from humans, but more practically, this understanding has been sought after so that we can quantify how much reasoning and inference our AI models are now capable of.
This step is also necessary so that we can secure them within appropriate guardrails. We now need to create intelligent automation services that we can define as “explainable AI” without bias or hallucinations, which means that intelligence can’t just be smart; it must be trusted and accountable.
NTT Research, Inc. is aiming to law down the laws of AI physics, economics, motion and mathematics with its newly formed Physics of Artificial Intelligence Group, a working body that has been spun off from a group within the NTT Research Physics & Informatics Lab. Underpinned by what the company calls an “interdisciplinary approach to understanding AI”, this initiative is led by NTT Research Scientist Dr. Hidenori Tanaka, a specialist in physics, neuroscience and machine learning.
Opening The AI Black Box
“As we stand today, we can mark a new step towards society’s understanding of AI through the establishment of NTT Research’s Physics of Artificial Intelligence Group,” NTT Research president and CEO Kazu Gomi said. “The emergence and rapid adoption of AI solutions across all areas of everyday life has had a profound impact on our relationship with technology. As AI’s role continues to grow, it is imperative we explore how AI makes people feel and how this can shape the advancement of new solutions. This new group aims to demystify concerns and bias around AI solutions to create a harmonious path forward for the coexistence of AI and humanity.”
From its early stages, the PHI Lab engineers say that they recognized the importance of understanding the “black box” nature of AI and machine learning to develop novel systems with drastically improved energy efficiency for computation. Rather similar to the way we experienced cloud computing for its power and flexibility factors first (and security came along somewhat shamefully second), we have gorged on the almost human-like intelligence of AI first… and then (again, perhaps somewhat shamefully) we have started to focus more adroitly on issues of trustworthiness and safety.
Biological & Artificial Intelligences, Plural
So how will the Physics of Artificial Intelligence Group uncover the insight needed to explain AI decisioning, reasoning and inference more clearly? The team says it will work in collaboration with academic researchers to address similarities between biological and artificial intelligences and unravel the complexities of AI mechanisms. The goal here is to work towards a more “harmonious fusion” of human and AI collaboration. That involves understanding how AI works in terms of how it is trained, how it accumulates knowledge and how it ultimately makes decisions.
According to NTT, “This approach echoes what physicists have done over many centuries: people had understood objects move when forces are applied, but it was physics that revealed the precise details of the relationship, which allowed humans to design machines we know today. For example, the development of the steam engine informed our understanding of thermodynamics, which in turn enabled the creation of advanced semiconductors. Similarly, the work of this group will shape the future of AI technology.”
To bring this whole project to fruition, the new group will continue to collaborate with the Harvard University Center for Brain Science.
What Is Neural Network Pruning?
Previous work by the academics involved here includes analyses focused on a neural network pruning algorithm. Not a new-age gardening technique for herbaceous borders, model “pruning” details the removal of less important, unimportant or extraneous parameters in a deep learning neural network (a machine learning network made up of neural nodes in the form of data values that are essentially interconnected, rather like the structure of our own brains). This process is undertaken to enable more efficient model inference. In practice, it is common for just the “weights” (importance ranking) of parameters to be pruned down rather than the biases, which govern the output of the neural nodes (also known as the activation function) as bias alteration has a more significant impact on the way machine learning delivers for AI.
We digress to denote and define (above) here for a reason. NTT Research is working to understand the minutiae of the internal mechanics of AI, so complete context is needed.
As part of its latest platform updates that have played out across NTT’s software-centric hardware line, the company has launched a new AI inference chip. As we know from this decade’s gravitation pull towards AI technologies, inference is the computing process where a “trained” AI model (that has previously been exposed to language, image or other models and also has the algorithmic logic in it to reason and rhyme wider meaning from the data it feeds on) is able to use that learning to make new predictions and inferences on raw unseen new data.
NTT’s new chip is known as a “large-scale integration” (sounds complicated, but it essentially just refers to the act of putting lots of transistors on a single chip to form integrated circuits, traditionally using silicon, but now also using light-based photonics processes) and so its huge power is suited to real-time AI inference processing of ultra-high-definition video up to 4K resolution and 30 frames per second.
Power-Constrained Deployments
This low-power technology is well suited to edge computing in internet of things deployments. It also works well for power-constrained terminal deployments in which conventional AI inferencing requires the compression of ultra-high-definition video for real-time processing.
In edge and power-constrained terminals (for example, sensors in manufacturing plants, retail point of sale systems or remote patient monitoring devices) devices are limited to power consumption by an order of magnitude lower than that of graphical processing units used in AI servers. Think about a power supply rating at around tens of watts in the former, compared to hundreds of watts in the latter. The LSI overcomes these restraints by implementing an NTT-created AI inference engine.
Executing the object detection algorithm You Only Look Once (known as YOLOv3 to its friends), this engine reduces computational complexity while ensuring detection accuracy, improving computing efficiency using interframe correlation and dynamic bit-precision control. Additionally, NTT researchers are collaborating with NTT DATA, Inc. on the advancement of this chip work in the area of proprietary attribute-based encryption technologies. Honest to the core (we hope), ABE enables fine-grained access control and flexible policy setting at the data layer, with shared-secret encryption technologies allowing for applications and data stores.
To define the above term, attribute-based encryption works to determine if data should be decrypted based on various attributes and policies, which are generally content-based, role-based and multi-authority access policies.
In terms of energy efficiency, other groups in the PHI Lab are already engaged in efforts to reduce the energy consumption of AI computing platforms through optical computing and a thin-film lithium niobate (the material used to build photonics chips based on light-based microprocessor design) technology. Inspired by the differential between watts consumed by LLMs and the human or animal brain, the new group will also explore ways to look more closely at the similarities between biological brains and artificial neural networks.
AI Mission: Harmonious With Humanity
Going forward, the Physics of Artificial Intelligence Group has a three-pronged mission:
a) It intends to deepen our understanding of the mechanisms of AI and integrate ethics from within, rather than through a patchwork of fine-tuning (which in AI circles we tend to call enforced learning)…. b) it will borrow from experimental physics and create systematically controllable spaces of AI and observe the learning and prediction behaviors… and c) it will aspires to heal the breach of trust between AI and human operators through improved operations and data control.
“The key for AI to exist harmoniously alongside humanity lies in its trustworthiness and how we approach the design and implementation of AI solutions,” said Dr. Tanaka. “With the emergence of this group, we have a path forward to understanding the computational mechanisms of the brain and how it relates to deep learning models. Looking ahead, our research hopes to bring about more natural intelligent algorithms and hardware through our understanding of physics, neuroscience and machine learning.”
What to think next then? If you take a walk down any given street in Tokyo, there are a patchwork of manhole-type utility covers on the sidewalks, many with the NTT logo emblazoned in cast iron on top. These grates hide a network of wires that the company has installed over the years to connect the city and form its neural network of information pathways. We might politely suggest then that the company already has something of a pedigree in terms of understanding how the guts of a technology subsystem works, whether it be a telephone network or an AI model.