Key AI Applications In Manufacturing
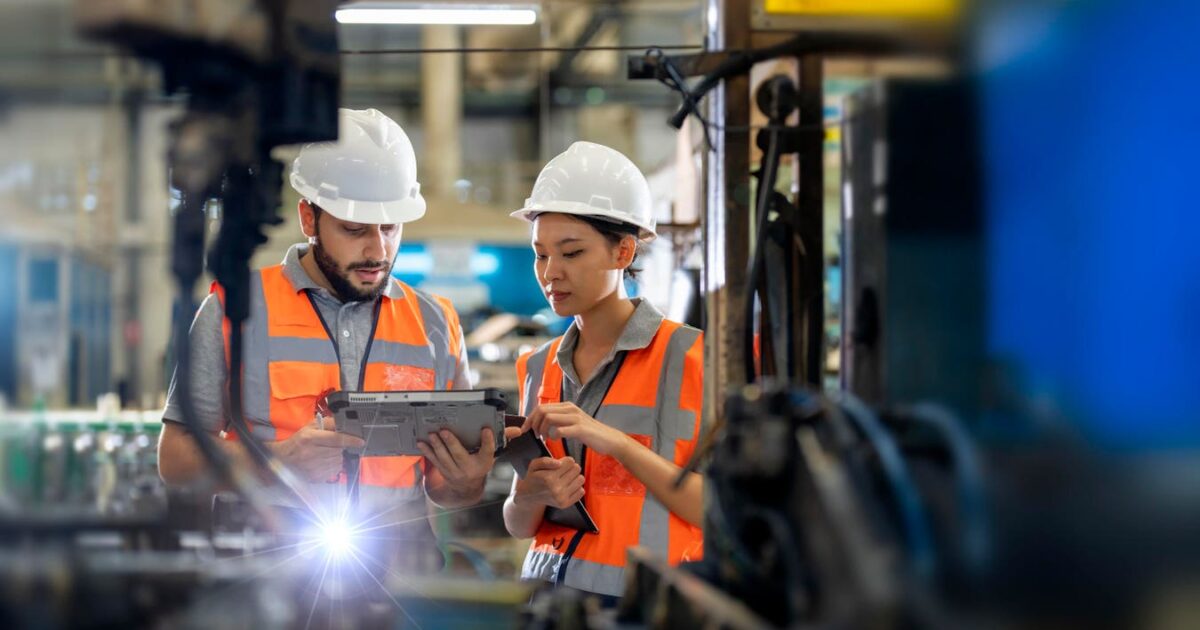
John Clemons is a Solution Consultant for Rockwell Automation. He’s been working in the field of Manufacturing Technology for over 30 years.
Artificial intelligence (AI) is making headlines with businesses and consumers much more so than in the manufacturing industry. But AI’s impact on manufacturing is huge, and while it may not be generating the headlines, it is generating bottom-line improvements for manufacturers, helping them reduce costs and increase their capabilities.
In the first two articles of this series, we examined the impact AI has on manufacturing and its real-world benefits. In this article, we’re going to look at some specific AI applications in manufacturing, what they mean for manufacturing and dig deeper into the benefits.
Data Collection
Manufacturing is fundamentally a data-driven process that relies on data at every step. Without the right data, mistakes occur and problems persist. Informed decisions based on accurate data can resolve problems and help avert future issues before they become problems.
AI tools are key to effective data collection and data management solutions in manufacturing. They offer robust data acquisition techniques from a wide range of data sources, including sensors, equipment, automation and control systems. AI makes sure the data is accurate, consistent, relevant and properly contextualized. Context is especially important as it provides the necessary background to understand and interpret the data effectively.
AI plays a crucial role in cleaning and preparing data for use by various information technology and operational technology (IT/OT) systems in manufacturing. Whether it’s automation and control systems, manufacturing execution systems/manufacturing operations management (MES/MOM) systems, enterprise resource planning (ERP) systems, product lifecycle management (PLM) systems, computerized maintenance management systems (CMMS), learning management systems (LMS) or quality management systems (QMS), these systems rely on high-quality data. AI helps ensure the data is clean, organized and has the relevant context before it’s provided to these systems.
AI is also key for cleaning and preparing data for other AI systems. Advanced analytics, machine learning (ML), deep learning and natural language processing (NLP) all require clean data to function effectively.
Machine Learning
ML is one of the cornerstones of AI in manufacturing. It has been used for over a decade and is often applied to individual pieces of equipment. The goal is to gather as much information as possible from equipment while it operates, then analyze and learn from that data to predict what’s going to happen.
In ML, AI processes large datasets, with the more data available, the better the learning process becomes. AI distinguishes between relevant and irrelevant data, identifying specific data patterns that can predict events that are likely to occur. With enough data and proper tuning, AI can achieve high accuracy with its predictions.
This capability’s value is apparent. Being able to predict equipment failures or quality issues is extremely valuable. Accurate predictions allow maintenance to be performed exactly when needed—not too early and not too late. In addition, understanding these predictions allows operators to avert quality problems before they arise. It also helps operators and managers implement continuous improvement plans to fine-tune and optimize the equipment and the entire manufacturing line.
Variations Of Machine Learning
Over the last decade, as ML has been implemented in many ways throughout manufacturing, some refinements have been made in the way that ML is undertaken. Here are some key variations:
• Supervised learning is a type of ML where good and bad data patterns are identified and used as the basis for learning. The AI tool is told which data patterns are good or bad and then learns to recognize those patterns, or similar ones, in the large amounts of data it processes. As more data becomes available, the AI tool improves its learning, becoming more sensitive to variations in the data, more adept at identifying good and bad patterns, and better able to detect spurious patterns. This process significantly enhances the algorithm’s accuracy.
• Unsupervised learning is ML where good and bad data patterns are either not identified or are not used as the basis for learning. The AI tool is learning to detect new data patterns. The AI algorithm learns how to find patterns in the data without having to learn from predefined examples. This type of learning is more difficult and time-consuming, requiring more data to get effective results. But it is highly valuable for detecting new patterns in data, which is especially important in systems that rely on pattern recognition and anomaly detection.
• Reinforcement learning is often applied in adaptive control systems. It allows machines, such as autonomous robots, to learn optimal actions through trial and error. This approach is useful throughout robotics and is used extensively in the latest generation of autonomous robots and in many autonomous systems used in manufacturing.
Natural Language Processing
NLP is exactly what it says. It’s using AI tools to analyze the language, making sure that the AI results are easy to understand. When people think of AI, NLP is what many people think of first.
NLP is especially valuable when it’s used with other AI tools. Consider the complexity of the tools mentioned previously and the vast amounts of data those tools process. Next, consider the potential complexity of the results in terms of the data, the predictions, and the prescriptions. NLP is especially valuable in manufacturing when it’s used to take those complex results and present them to the operators, the supervisors or the engineers in ways that are easy to understand and are clearly actionable.
Generative AI
Generative AI is beginning to have a significant impact in manufacturing. There’s so much to cover on this topic that the next several articles in this series will focus on generative AI.
Conclusion
AI applications in data collection, ML and NLP are becoming a mainstay in manufacturing. We’ve barely scratched the surface in the application of these technologies in manufacturing.
Manufacturing is a fertile ground for AI applications. AI is already helping manufacturers around the world reduce costs, increase capabilities and generate significant bottom-line returns—and we’re just getting started.
Forbes Technology Council is an invitation-only community for world-class CIOs, CTOs and technology executives. Do I qualify?