Why Breaking Data Silos Is Key
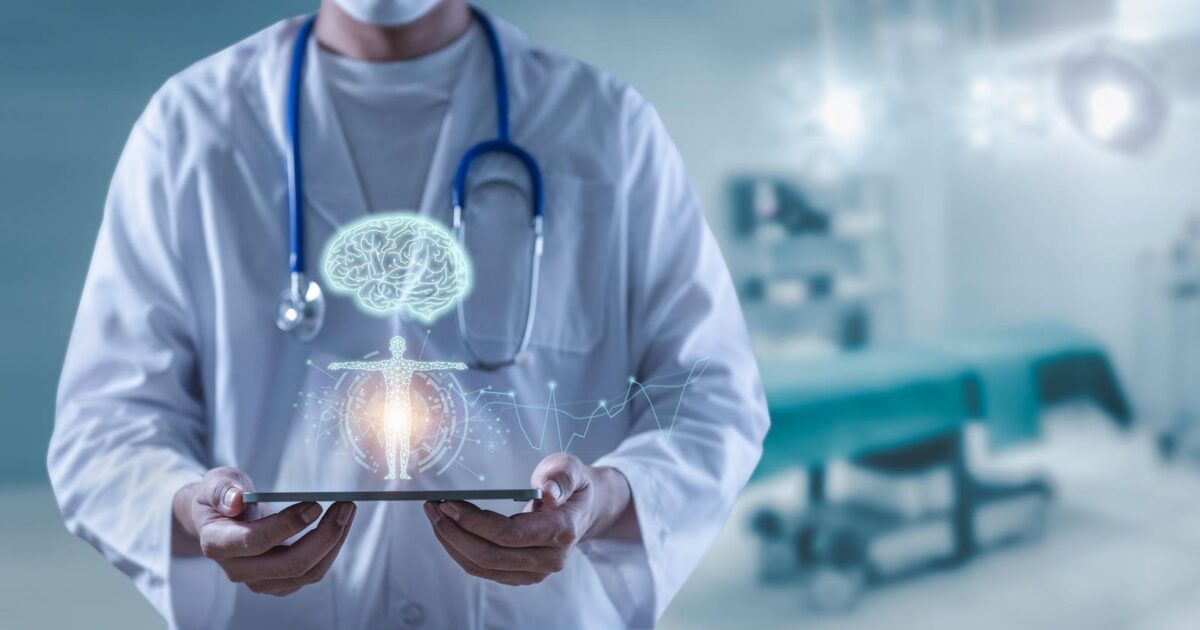
Sanjay Juneja, M.D., Co-Founder of TensorBlack Inc.
Artificial intelligence (AI) is rapidly transforming medicine, promising to revolutionize diagnostics, treatment planning and operational efficiency. But there’s a critical—and often overlooked—flaw in many AI-driven healthcare models: They are only as good as the data they are trained on.
For AI to truly improve and standardize healthcare delivery, we must confront a fundamental issue—the limited and often siloed nature of clinical data. If institutions and health systems continue to train AI models solely within their own populations and geographic regions, they risk developing highly performant yet ultimately narrow-scope solutions. These models may excel in their specific environments but falter when applied broadly, leading to skepticism, reduced adoption and even potential harm due to unrecognized biases.
To create AI models that are generalizable, equitable and ultimately trusted, we must embrace a more collaborative, open-minded approach to data sharing across diverse geographies and demographics. Without this shift, AI in healthcare remains vulnerable to criticism—and its potential impact will be stunted.
Why Institutional Data Silos Undermine AI’s Potential
Even the most sophisticated AI models are only as representative as the data they ingest. Training an algorithm on patient data from a single institution—or even a small network of institutions—introduces an inherent bias based on a variety of unpredictable variables, including:
Institutional Ordering Behavior
Physicians at different hospitals have unique practice patterns, influenced by institutional culture, preferred diagnostic pathways and local policies. An AI model trained at one institution may not perform optimally when deployed elsewhere because its recommendations are shaped by the preferences of a particular clinical environment.
Genetic And Enzymatic Variability
Populations in different geographic regions have distinct genetic predispositions and enzymatic tendencies due to single nucleotide polymorphisms (SNPs) that impact drug metabolism, disease prevalence and treatment responses. A model trained primarily on a Midwestern U.S. population, for instance, may not generalize well to patients in Southeast Asia or even to different ethnic groups within the U.S.
Lifestyle-Driven Health Variables
A patient in Boulder, Colorado, with an active outdoor lifestyle and lower BMI metrics may have a vastly different baseline for health than a sedentary population in Baton Rouge, Louisiana, where dietary habits and obesity prevalence are higher. These lifestyle-driven differences impact disease progression, treatment response and predictive modeling, yet most AI training datasets fail to account for them.
When AI models fail to generalize, their acceptance among clinicians and institutions is compromised. If a model trained on a predominantly Caucasian, urban population struggles when applied to a rural or ethnically diverse demographic, concerns over bias and fairness grow. And if these concerns aren’t proactively addressed, AI adoption in healthcare faces significant hurdles—regardless of how technically advanced the models become.
The Danger Of Overfitting AI To Localized Data
In machine learning, overfitting occurs when a model learns patterns too closely tied to its training data, making it perform well in that context but poorly elsewhere. In healthcare AI, localized overfitting isn’t just a technical limitation—it’s a matter of patient safety and trust.
Imagine an AI-driven cancer risk prediction tool trained at a single comprehensive cancer center in an urban region. The tool might perform with remarkable accuracy for patients seen at that institution. But when deployed at a community hospital serving a different socioeconomic population, the model’s effectiveness may plummet due to missing contextual factors—such as differences in health literacy, access to preventive care or environmental exposures that weren’t captured in the original dataset.
The inevitable result? Physicians lose confidence in AI recommendations, regulatory scrutiny increases, and adoption stagnates. This is already happening with some AI-based radiology tools that demonstrate strong performance at the institutions where they were trained but struggle when tested in broader, real-world settings.
Without cross-institutional and cross-regional data sharing, AI risks becoming a fragmented patchwork of solutions—each optimized for its own niche, yet failing to deliver the widespread transformation that healthcare desperately needs.
A Collaborative Data Approach: The Path To Generalizability And Trust
To move AI from promising to indispensable in medicine, we must shift from institutionally confined AI training to a more holistic, multi-source and globally inclusive data strategy. This requires:
1. Expanding Cross-Institutional Data Sharing
Health systems, academic centers and industry leaders must develop frameworks for responsibly sharing de-identified patient data across institutions, ensuring that AI models are exposed to a broader range of patient demographics, disease presentations and clinical workflows. Federated learning—a technique that enables AI models to train on decentralized datasets without directly sharing sensitive patient data—offers a promising approach to overcoming privacy concerns while increasing data diversity.
2. Prioritizing Geographically And Ethnically Diverse Training Sets
AI models should not just be tested but actively trained on populations that reflect real-world diversity. This includes integrating data from urban, suburban and rural populations, ensuring representation across racial and ethnic groups, and accounting for socioeconomic and lifestyle differences that impact health outcomes.
3. Regulatory And Policy Support For Ethical AI Development
Governments and regulatory bodies must play a role in incentivizing collaborative AI development. This could include funding for multi-institutional AI research initiatives, policy frameworks that encourage responsible data sharing and standardized evaluation metrics that emphasize generalizability.
4. Transparent Validation And Bias Audits
Every AI model deployed in clinical practice should undergo rigorous external validation on datasets that were not part of its initial training. Routine bias audits should be conducted to assess model performance across different patient populations, ensuring fairness and mitigating the risk of AI-driven healthcare disparities.
The Bigger Picture: AI As A Global Healthcare Standardizer
The true promise of AI in healthcare is not just automation or efficiency—it’s the ability to standardize and improve care across all regions, regardless of geography, institution or socioeconomic factors. But this can only be realized if AI is trained on diverse, representative data that reflects the full spectrum of human health.
If we fail to embrace collaboration of these potentially transformative tools, we risk not only ineffective models but also a crisis of trust—one that could delay, or even derail, AI’s integration into mainstream healthcare. Medicine is universal, and our data should be too.
Forbes Technology Council is an invitation-only community for world-class CIOs, CTOs and technology executives. Do I qualify?