AI’s Magic Cycle
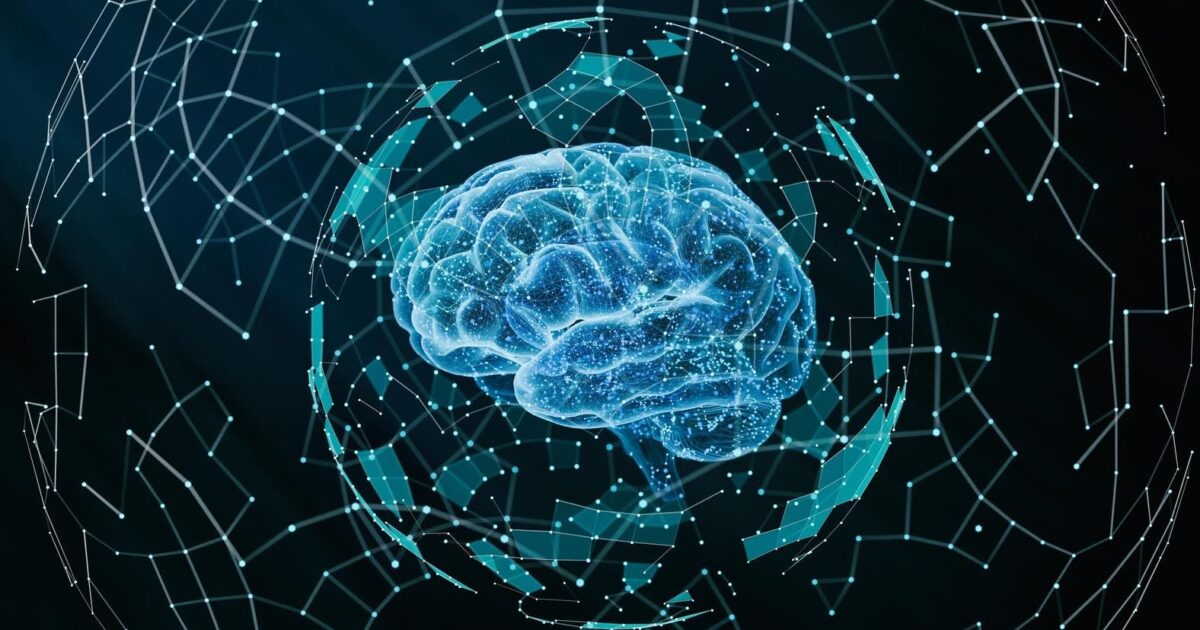
Linkedin: Here’s some of what innovators are thinking about with AI research today
Artificial Intelligence concept – 3d rendered image.
When people talk about the timeline of artificial intelligence, many of them start in the 21st century.
That’s forgivable if you don’t know a lot about the history of how this technology evolved. It’s only in this new millennia that most people around the world got a glimpse of what the future holds with these powerful LLM systems and neural networks.
But for people who have been paying attention and understand the history of AI, it really goes back to the 1950s.
The Dartmouth Conference and Beyond
In 1956, a number of notable computer scientists and mathematicians met at Dartmouth to discuss the evolution of intelligent computation systems.
And you could argue that the idea of artificial intelligence really goes back much further than that. When Charles Babbage made his analytical engine decades before, even rote computation wasn’t something that machines could do.
But when the mechanical became digital, and data became more portable in computation systems, we started to get those kinds of calculations and computing done in an automated way.
Now there’s the question of why artificial intelligence didn’t come along in the 1950s, or in the 1960s, or in the 1970s.
“The term ‘Artificial Intelligence’ itself was introduced by John McCarthy as the main vision and ambition driving research defined moving forward,” writes Alex Mitchell at Expert Beacon. “65 years later, that pursuit remains ongoing.”
What it comes down to, I think most experts would agree, is that we didn’t have the hardware. In other words, you can’t build human-like systems when your input/output medium is magnetic tape.
But in the 1990s, the era of big data was occurring, and the cloud revolution was happening. And when those were done, we had all of the systems we needed to host LLM intelligence.
Just to sort of clarify what we’re talking about here, most of the LLMs that we use work on the context of next-word or next-token analysis – they’re not sentient, per se, but they’re using elegant and complex data sets to mimic intelligence.
And to do that, they need big systems. That’s why the colossal data centers are being built right now, and why they require so much energy, so much cooling, etc.
The Magic Cycle of Research
At an Imagination in Action event this April, I talked to Yossi Mathias, a seasoned professional with 19 years at Google who is the head of research at Google, about research there and how it works.
He talked about a cycle for a research motivation that involves publishing, vetting and applying back to impact.
But he also spoke to that idea that AI really goes back father than most people think.
“It was always there,” he said, invoking the idea of the Dartmouth conference and what it represented. “Over the years, the definition of AI has shifted and changed. Some aspects are kind of steady. Some of them are kind of evolving.”
Then he characterized the work of a researcher, to compare motives for groundbreaking work.
“We’re curious as scientists who are looking into research questions,” he said, “but quite often, it’s great to have the right motivation to do that, which is to really solve an important problem.”
Looking Into Societal-Centered AI
“Healthcare, education, climate crisis,” he continued. “These are areas where making that progress, scientific progress …actually leads into impact, that is really impacting society and the climate. So each of those I find extremely rewarding, not only in the intellectual curiosity of actually addressing them, but then taking that and applying it back to actually get into the impact that they’d like to get.”
Ownership of a process, he suggested, is important, too.
“An important aspect of talking about the nature of research at Google is that we are not seeing ourselves as a place where we’re looking into research results, and then throwing them off the fence for somebody else to pick up,” he said. “The beauty is that this magic cycle is really part of what we’re doing.”
He talked about teams looking at things like flood prediction,where he noted to so potential for future advancements.
We also briefly went over the issue of quantum computing,where Mathias suggested there’s an important milestone ahead.
“We can actually reduce the quantum error, which is one of the hurdles, technological hurdles,” he said. “So we see good progress, obviously, on our team.”
One thing Mathias noted was the work of Peter Shore, whose algorithm, he suggested, demonstrated some of the capabilities that quantum research could usher in.
“My personal prediction is that as we’re going to get even closer to quantum computers that work, we’re going to see many more use cases that we’re not even envisioning today,” he noted.
People Coming Together
Later, Mathias spoke about his notion that AI should be assistiveto humans, and not a replacement for human involvement.
“The fun part is really to come together, to brainstorm, to come up with ideas on things that we never anticipated coming upwith, and to try out various stuff,” he said.
Explaining how AI can fill in certain gaps in the scientific process, he described a quick cycle by which, by the time a paper is published on a new concept, that new concept can already be in place in, say, a medical office.
“The one area that I expect actually AI to do much more (in) is really (in) helping our doctors and nurses and healthcare workers,” Mathias said.
Working Toward the Future
I was impressed by the scope of what people have done, at Google and elsewhere.
So whether it’s education or healthcare or anything else, we’re likely to see quick innovation, and applications of these technologies to our lives. And that’s what the magic cycle is all about.