Building Enterprise AI On A Granite Foundation Of Trust And Confidence
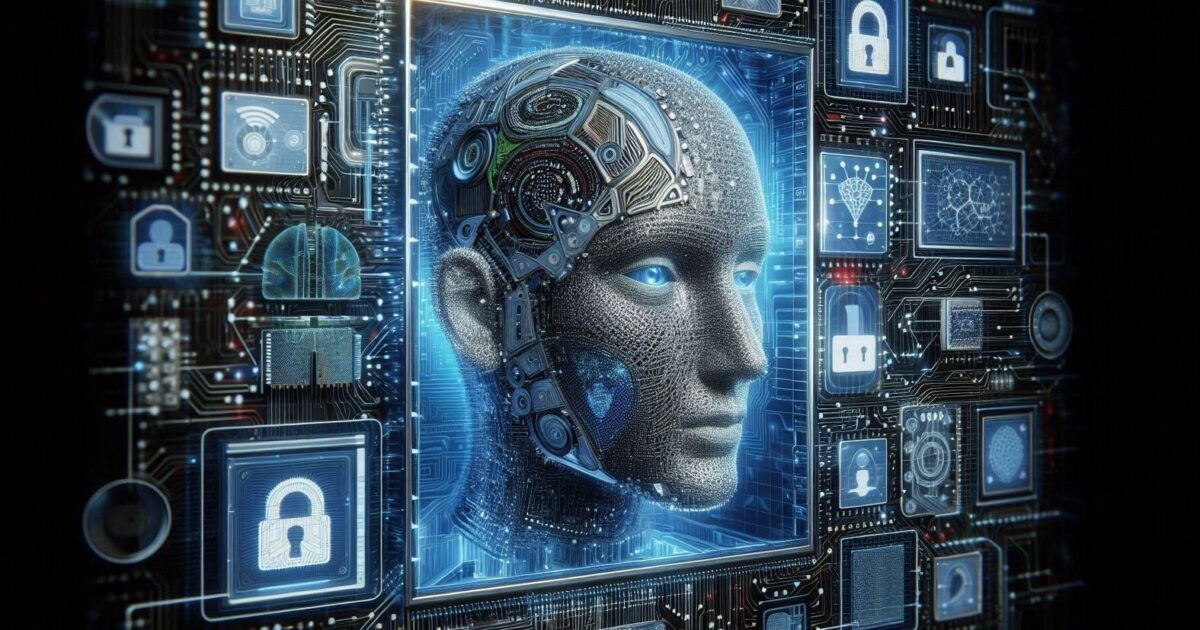
Safety and Security in Enterprise AI
While inference cost and performance are critical factors in enterprise AI, they are not the only considerations. Enterprises must also ensure their AI models can withstand stringent security, privacy, and regulatory compliance requirements. At the heart of a robust AI strategy lies the ability to develop and maintain trust and confidence in the AI systems being deployed. This means that beyond optimizing for cost and performance, businesses need to prioritize the integrity and security of their models. Ensuring that AI systems are protected with adequate guardrails, compliant with data protection regulations and can secure sensitive information is paramount.
When enterprises assess which foundation models should be used as part of their AI implementation, it is this holistic approach that should be applied. Consider, for example, some models that were recently released with the latest chain of thought reasoning capabilities. On one hand are Qwen 2.5 7B and Llama 3.1 8B, two models distilled by DeepSeek using DeepSeek R1, and on the other, IBM Granite 3.2. With DeepSeek having distilled the former two models from its R1 model, any analysis of those models in terms of safety and security, must first start with an assessment of DeepSeek R1.
DeepSeek took the world by storm upon its release of its latest model, DeepSeek R1, having demonstrated that it is possible to train high-performing models at a fraction of the cost traditionally associated with such endeavors. This cost-efficiency extends to its usage for inference, making it an economically attractive option for businesses looking to leverage AI.
However, despite its notable advancements in performance and cost efficiency, DeepSeek R1 has been found to possess critical vulnerabilities. In one study conducted by Cisco, DeepSeek R1 was found to be susceptible to jailbreak attacks in 100% of attempts made during the study. It also has a strong China bias with certain topics like previous political uprisings being suppressed.
Granite 3.2, is a family of models developed by IBM which consists of language models, time series models, geospatial models, embedding models, speculative decoding models, guardian models and with this latest 3.2 release, vision models.
Granite 3.2 Family Updates
Similar to the DeepSeek R1-distilled Qwen 2.5 7B and Llama 3.1 8B, Granite 3.2, incorporates reasoning capabilities into its language models. However, unlike the DeepSeek-based models, IBM asserts that their approach developing Granite 3.2 maintains safety and robustness while still delivering reasoning performance.
AttaQ Benchmark Results
The chart above shows results from tests conducted by IBM using the AttaQ benchmark. This benchmark utilizes approximately 1,400 questions across categories such as deception, discrimination, harmful information, substance abuse, or sexual content among others, to prompt a target model and assess its tendency to generate harmful, inaccurate or undesirable responses. The data above shows that when utilizing DeepSeek R1 to impart reasoning capabilities onto smaller models such as Qwen 2.5 7B and Llama 3.1 8B, safety and robustness are negatively impacted, while IBMs implementation of chain-of-thought reasoning maintained the integrity of its results.
In addition to this inherent approach to maintaining safety when implementing reasoning, as part of its Granite family of models, IBM also provides companion guardrail models called Granite Guardian, to further improve on the built-in performance and to help enterprises implement guardrails alongside any model of choice, not just Granite models. First introduced last year as part of IBMs initial Granite 3.0 release, Granite Guardian assesses both input prompts and output responses for bias, safety, and hallucinations. More specifically, the models are trained to detect jailbreaking, bias, violence, profanity, sexual content, unethical behavior and both RAG and function calling hallucinations. While these capabilities were also available in the previous versions, the latest 3.2 release, according to IBM, maintains the same performance but does so using up to 40% less active parameters. This reduction in parameters significantly improves both cost and latency.
On the subject of costs, DeepSeek R1 is approximately $2.19 per million output tokens through its API, Granite models running on watsonx.ai range from $0.10 to $0.20 per million output tokens, an order of magnitude less while still delivering on the trusted nature of IBMs models. These costs are for models deployed through a cloud provider. When deployed locally, costs will vary based on the on-prem setup. However, the cloud provider deployment costs can serve as a good proxy to indicate relatively how much investment will be required to scale on-prem implementations.
These factors don’t necessarily mean you can’t or shouldn’t use DeepSeek if it’s the right tool for the job. However, they do underscore the necessity for a comprehensive risk assessment and management framework that considers cost, performance, security, safety, and data and training compliance when selecting models on which to base enterprise AI systems. In most applications, enterprises must prioritize robust security measures, regular compliance evaluations, and ongoing monitoring to mitigate the inherent risks associated with implementing such systems. Assessing total cost of ensuring operational integrity should be the determining factor as opposed to simply cost per token.
Tirias Research tracks and consults for companies throughout the electronics ecosystem from semiconductors to systems and sensors to the cloud. Members of the Tirias Research team have consulted for IBM, Nvidia, AMD and other companies throughout the server, AI and Quantum ecosystems.