Factoring Data Streaming Considerations Into Your Business’ AI Strategy
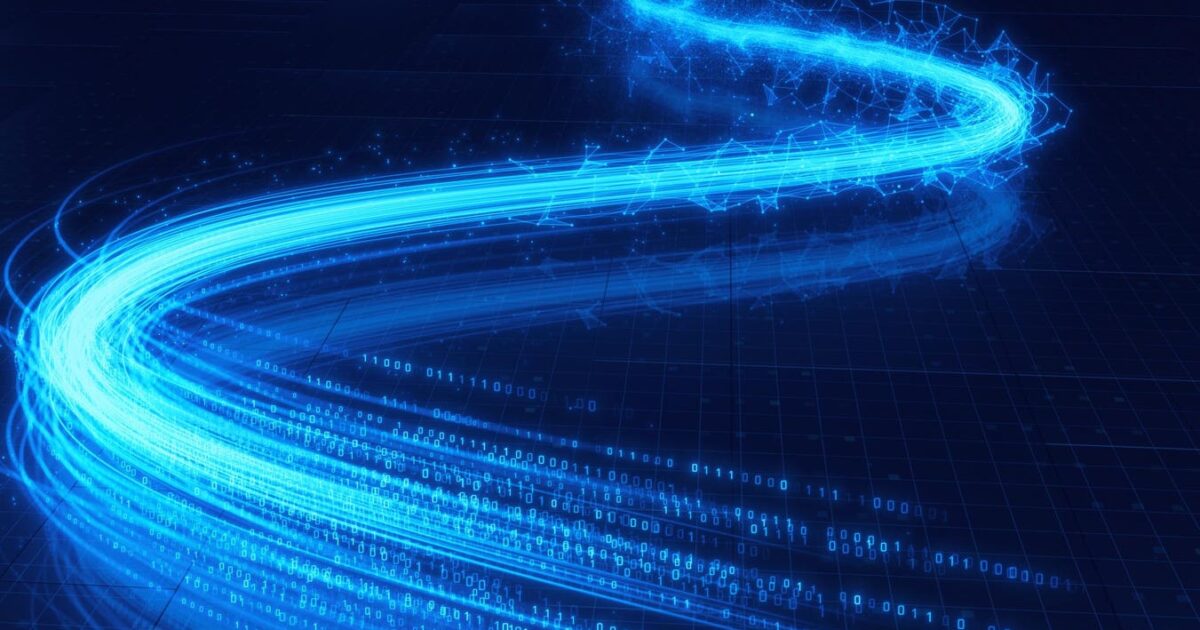
Guillaume Aymé is CEO of Lenses.io, a pioneer in the streaming market that has transformed the way engineers work with real-time data.
AI’s adoption rate is expected to dramatically accelerate in the year ahead, with businesses turning to novel applications like AI agents to improve agility and find competitive advantages. One way to get the most out of this shift is through real-time data streaming, which boosts AI’s capabilities. AI is only as good as the data feeding it, and businesses are turning to unfiltered data provided in real time as one option to help provide the high-quality data they need.
But not all businesses are currently built for real-time data streaming. To gain a competitive advantage in our increasingly digital and interconnected world, businesses must develop new architectures that enable better agility, advanced autonomous operations and simplified application development.
Building Agility For Market Readiness
An executive recently told me that their business was having trouble with volatility. “We don’t know what horse to bet on,” they said. I wasn’t surprised, considering the recent financial turbulence, geopolitical situations, regulatory landscape and disruption caused by open-source AI models.
Building agility is necessary for market readiness, which businesses should assume will be needed because disruption, in one way or another, will be experienced at some point. Leaders should build this understanding into their data and AI strategies, putting in place the tools and practices that offer maximum operational flexibility to respond to the market as quickly as possible.
The ability to consume a common stream of data and respond in real time can enable agility and empower engineers to build new applications addressing use cases that would otherwise not have been possible, such as fraud and algorithmic trading. But businesses should ensure they have the flexibility to move or distribute real-time data across architectures, environments and vendor technologies to respond to the uncertainty in the market. This will grow increasingly important as AI is deployed in more and newer ways.
Thankfully, open source and standards like Apache Iceberg and Kafka API are making it easier for businesses that are behind to catch up.
AI Agents Are The New Microservices
Businesses have the opportunity to architect AI agents and large language model (LLM)-based applications as event-driven applications (EDAs). This is how modern software architecture has been designed for over 10 years—with an emphasis on smaller, discrete “microservices” being decoupled from each other but communicating through middleware infrastructure.
Drag-and-drop tools (such as Longflow) for building agentic flows have simplified the work of engineers building powerful agentic flows and embedding them into software applications. But like microservices, it’s critical to ensure each agent isn’t given too much responsibility.
Therefore, application and IT teams should start thinking about how agents will be integrated into an IT landscape at scale. Event-driven architectures are simpler to scale and help prepare for a future where numerous agents, potentially even hundreds of thousands, will be deployed for global enterprises. This architecture allows agents to “speak” to each other through real-time data sharing, which opens up new use cases for business automation.
Consider retail security cameras connected to an agent that detects a theft in an outlet. This system can’t afford data latency, and by being able to communicate with multiple downstream systems (themselves AI agents), the theft could be prevented in the form of an alarm sounding and law enforcement being called.
Once businesses grow comfortable with having AI agents collaborate, they’ll create new possibilities for improved capabilities provided by true automation.
Unifying Real-Time Application And AI Development
Little separates AI, software and data applications today. Historically, these applications and the teams behind them have been separated, adopting different practices, tools and architectures, and even operating in different cloud environments. For businesses to be successful in fostering collaboration and building real-time data pipelines to enable AI, engineers—and even business users—need to be equipped with self-service tooling.
Many large enterprises already understand the importance of the unified experience. The businesses that don’t follow suit will be left behind, unable to get the most out of AI.
Forbes Technology Council is an invitation-only community for world-class CIOs, CTOs and technology executives. Do I qualify?