Gender Bias In AI: Addressing Technological Disparities
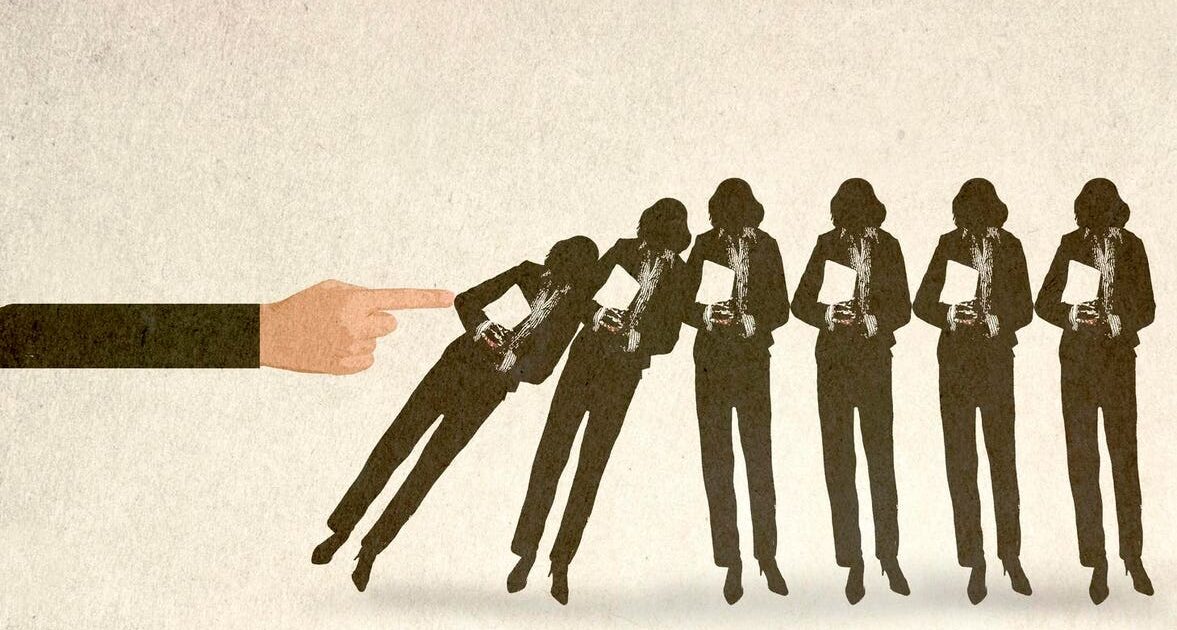
Swati Tyagi is a seasoned AI/ML professional and has made significant contributions to gender equity in AI and explainable credit scoring.
Artificial intelligence (AI) is reshaping our world at an unprecedented pace, automating decisions across healthcare, employment, criminal justice and finance. However, as these systems become more integrated into our daily lives, a concerning pattern has emerged: Many AI systems demonstrate significant gender bias, particularly disadvantaging women and women of color. This bias threatens to reverse hard-won progress toward gender equality if left unchecked. This article examines the origins of AI bias and its consequences across different sectors and proposes actionable solutions to create more equitable AI systems.
Understanding The Origins Of AI Bias
AI systems don’t develop biases spontaneously—they learn them from the data they’re trained on. These systems analyze enormous datasets that frequently reflect historical inequalities and societal prejudices. When training data overrepresents male experiences, particularly those of white men, the resulting algorithms inevitably produce biased outcomes.
The problem becomes particularly troubling with the increasing use of synthetic data. Although artificially generated data can help overcome data shortages, it presents unique challenges. Synthetic data created from already-biased algorithms simply amplifies existing prejudices. Additionally, such data often lacks transparency, making it difficult to identify and address embedded biases.
This creates a dangerous feedback loop: Biased AI systems make discriminatory decisions that generate more biased data, which trains even more biased systems. As AI becomes increasingly embedded in decision-making processes across sectors, this cycle threatens to systematically exclude women from opportunities and reinforce gender stereotypes.
Real-World Impacts On Women’s Lives
Healthcare Disparities
In healthcare, AI bias manifests in diagnostic and treatment discrepancies. AI diagnostic tools often show lower accuracy rates for women, particularly women of color. These systems frequently misdiagnose conditions or recommend inappropriate treatments because they were primarily trained on data from male patients. Such biases have serious consequences for women’s health outcomes, including maternal health, where existing disparities are already pronounced.
Employment Barriers
AI recruitment tools frequently exhibit what experts call the “mom penalty”—systematically disadvantaging women who have taken career breaks for childcare. These systems often interpret employment gaps as negative indicators rather than recognizing the valuable skills developed during caregiving periods. Additionally, AI hiring algorithms tend to favor candidates with traits historically associated with male leadership, further limiting women’s advancement opportunities in traditionally male-dominated fields like technology and executive management.
Financial Disadvantages
The finance sector’s increasing reliance on AI for credit decisions has created new barriers for women seeking financial independence. AI lending algorithms frequently offer women less favorable terms or outright deny them credit, even when comparing applicants with identical financial profiles. These practices limit women’s ability to secure capital for education, homeownership or entrepreneurship—all crucial pathways to building financial security.
Criminal Justice Inequities
In criminal justice, algorithmic risk assessment tools have shown concerning bias against women of color. These systems disproportionately classify minority women as higher risk, potentially leading to harsher sentences and prolonged incarceration. This algorithmic discrimination compounds existing racial and gender disparities within the justice system, creating devastating consequences for affected women and their families.
Comprehensive Solutions For Equitable AI
Addressing gender bias in AI requires coordinated efforts across multiple sectors:
Improving Data And Development Practices
• Diverse Training Data: AI developers must prioritize collecting diverse, representative datasets that include experiences across gender, race and other demographic factors.
• Regular Bias Auditing: Organizations should implement mandatory bias testing before deployment and continue monitoring systems after implementation.
• Responsible Synthetic Data Usage: When using synthetic data, developers must implement rigorous safeguards to prevent the amplification of existing biases.
• Diverse Development Teams: AI companies should prioritize building diverse technical teams, as homogeneous groups are more likely to overlook potential biases.
Sector-Specific Interventions
Healthcare
• Require clinical validation of AI diagnostic tools across diverse populations.
• Develop patient-centered design approaches that incorporate diverse perspectives.
• Mandate transparency in how AI systems reach medical conclusions.
Employment
• Require disclosure of evaluation criteria used in AI hiring tools.
• Develop mechanisms for candidates to contest potentially biased decisions.
• Design recruitment algorithms that recognize diverse career paths.
Finance
• Implement regulatory frameworks requiring fairness testing in lending algorithms.
• Develop alternative credit evaluation methods that recognize different financial patterns.
• Create transparency requirements for AI-driven financial decisions.
Policy And Regulatory Frameworks
Strong regulatory oversight is essential for ensuring AI systems promote rather than undermine gender equality:
• Develop comprehensive AI fairness standards with specific provisions addressing gender bias.
• Require impact assessments before deploying AI in sensitive domains.
• Implement penalties for organizations that deploy discriminatory systems.
• Create intersectional policies that address the unique challenges faced by women from various backgrounds.
Education And Advocacy
• Expand AI literacy programs targeting women and underrepresented groups.
• Support organizations promoting women in technology.
• Advocate for sustainable funding for diversity initiatives in tech.
• Build coalitions between technical experts and gender equality organizations.
Conclusion
The rise of AI presents both promise and peril for gender equality. Without deliberate intervention, AI systems risk entrenching existing inequalities and creating new forms of discrimination against women. However, with thoughtful development practices, robust oversight and inclusive design, AI can become a powerful tool for creating a more equitable society.
The moment for action is now. As AI systems become more deeply integrated into critical decision-making processes, addressing gender bias must be a priority. By implementing comprehensive solutions across technical, organizational and policy domains, we can help ensure that AI advances rather than impedes progress toward gender equality.
Forbes Technology Council is an invitation-only community for world-class CIOs, CTOs and technology executives. Do I qualify?