Mollick Presents The Meaning Of New Image Generation Models
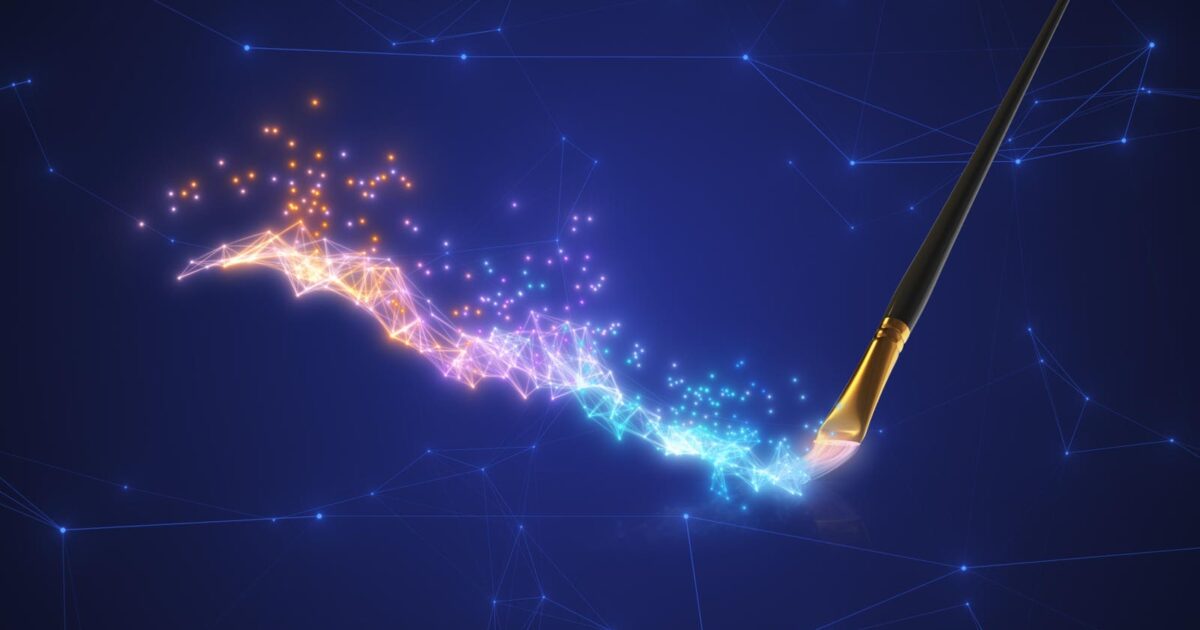
Paintbrush dynamically illustrates the innovative concept of generative AI art. This mesmerizing … More
What does it mean when AI can build smarter pictures?
We found out a few weeks ago as both Google and OpenAI unveiled new image generation models that are fundamentally different than what has come before.
A number of important voices chimed in on how this is likely to work, but I didn’t yet cover this timely piece by Ethan Mollick at One Useful Thing, in which the MIT graduate looks at these new models in a detailed way, and evaluates how they work and what they’re likely to mean to human users.
The Promise of Multimodal Image Generation
Essentially, Mollick explains that the traditional image generation systems were a handoff from one model to another.
“Previously, when a Large Language Model AI generated an image, it wasn’t really the LLM doing the work,” he writes. “Instead, the AI would send a text prompt to a separate image generation tool and show you what came back. The AI creates the text prompt, but another, less intelligent system creates the image.”
Diffusion Models Are So 2021
The old models also mostly used diffusion to work.
How does diffusion work?
The traditional models have a single dimension that they use to generate images.
I remember a year ago I was writing an explanation for an audience of diffusion by my colleague Daniela Rus, who presented it at conferences.
It goes something like this – the diffusion model takes an image, introduces noise, and abstracts the image, before denoising it again to form a brand new image that resembles what the computer already knows from looking at images that match the prompt.
Here’s the thing – if that’s all the model does, you’re not going to get an informed picture. You’re going to get a new picture that looks like a prior picture, or more accurately, thousands of pictures that the computer saw on the Internet, but you’re not going to get a picture with actionable information that’s reasoned and considered by the model itself.
Now we have multimodal control, and that’s fundamentally different.
No Elephants?
Mollick gives the example of a prompt that asks the model to create an image without elephants in the room, showing why there are no elephants in the room.
Here’s the prompt: “show me a room with no elephants in it, make sure to annotate the image to show me why there are no possible elephants.”
When you hand this to a traditional model, it shows you some elephants, because it doesn’t understand the context of the prompt, or what it means. Furthermore, a lot of the text that you’ll get is complete nonsense, or even made-up characters. That’s because the model didn’t know what letters actually looked like – it was getting that from training data, too.
Mollick shows when you hand the same prompt to a multimodal model. It gives you exactly what you want – a room with no elephants, and notes like “the door is too small” showing why the elephants wouldn’t be in there.
Challenges of Prompting Traditional Models
I know personally that this was how the traditional models worked. As soon as you asked them not to put something in, they would put it in, because they didn’t understand your request.
Another major difference is that traditional models would change the fundamental image every time you ask for a correction or a tweak.
Suppose you had an image of a person, and you asked for a different hat. You might get an image of an entirely different person.
The multimodal image generation models know how to preserve the result that you wanted, and just change it in one single small way.
Preserving Habitats
Mollick gives another example of how this works: he shows an otter with a particular sort of display in its hands. Then the otter appears in different environments with different styles of background.
This also shows the detailed integration of multi Moto image generators.
A whole pilot deck.
For a used case scenario BB shows how you could take one of these multimodal models and have it designed an entire pitch deck for guacamole or anything else?
All you have to do is say come up with this type of deck and the model will get right to work looking at what else is on the Internet, Synthesizing it and giving you the result.
As Mick mentions this will make all sorts of human work obsolete very quickly.
We will need well considered framework