Nvidia Partners With Swedish Consortium: AI Factory Redefines Economy
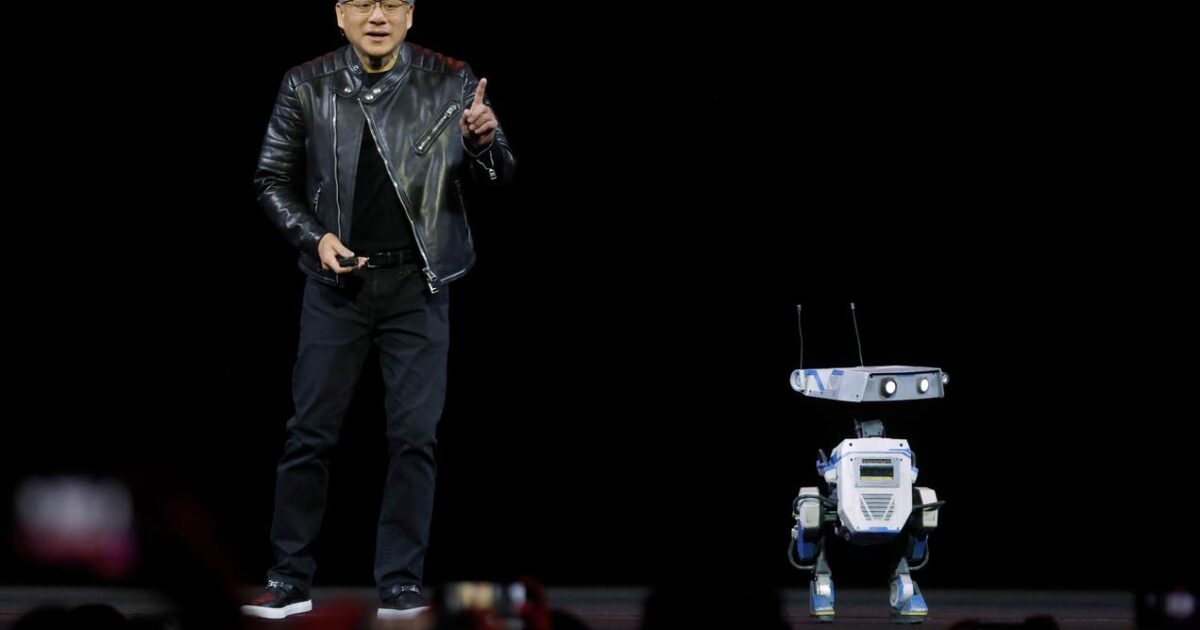
SAN JOSE, CALIFORNIA – MARCH 18: Nvidia CEO Jensen Huang delivers the keynote address with a robot … More
AI is touching every corner of enterprise activity, from autonomous vehicles and robotics industries to drug discovery and finance. This makes the compute infrastructure essential for any economy and company competitiveness increasingly shaped by AI technologies. Countries are also racing to make plans to build AI infrastructure to sustain business growth and innovation.
In Sweden, a consortium of Ericsson, AstraZeneca, Saab, and SEB just announced collaborations with Nvidia to build an AI factory powered by the latest Grace Blackwell GB 300 systems. In Europe, the EU’s AI Factory initiative, backed by the EuroHPC Joint Undertaking, is creating a network of at least 15 AI-optimized supercomputing hubs and several AI Gigafactories to support startups, SMEs, and research institutions across sectors like healthcare, climate, and manufacturing across Europe. In the U.S., Nvidia has announced it will manufacture AI supercomputers domestically for the first time, tapping partners like TSMC, Wistron, Amkor, and SPIL to produce up to $500 billion in AI infrastructure over the next four years. Like wise, in Asia, private and public sectors alike are investing in building AI factories with an emphasis on business innovation and training AI talents.
The era of AI factory has arrived, bringing with it economic opportunities and enhanced productivity but also safety risks and energy challenges, and the dwindling of tangible human connections.
Why Enterprises Need AI Factories
Enterprises across sectors are integrating AI into their research and development. At Pfizer, AI and supercomputing were instrumental in the development of PAXLOVID, the company’s COVID-19 oral treatment. AI accelerated molecule discovery, reduced clinical data analysis time by 50%, and cut production cycle time by 67%. In collaboration with Nvidia and Classiq, BMW is exploring how quantum-AI synergies can enhance vehicle engineering and manufacturing logistics. Similarly, Goldman Sachs uses LLMs to streamline legal and compliance operations, while Dell’s enterprise clients are leveraging agentic AI to deploy digital workers across cloud and edge environments. Goldman Sachs has rolled out its GS AI Assistant to over 10,000 employees—bankers, traders, and asset managers alike. Designed to evolve from simple task automation (proofreading, code translation) to agentic behavior, the system is trained to act like a seasoned Goldman employee—consulting the right data, using appropriate algorithms, and reflecting the firm’s values and decision-making protocols.
As computing becomes embedded into daily workflows, drafting documents, generating code, performing statistical modeling, even designing new products, enterprise demand for compute is exploding. McKinsey has reported that over the next three years, 92 percent of companies plan to increase their AI investments. To keep up, organizations need not just more GPUs, but entire supply chains that deliver compute at scale, speed, and security.
This is where AI factories come in. Unlike traditional data centers or chip fabs, AI factories fuse three critical layers: chip production and packaging, supercomputer system assembly, and data center-scale deployment. Dell Technologies, for example, in collaboration with Nvidia, has launched Dell AI Factory 2.0, an end-to-end enterprise platform tailored for agentic AI, enabling federated data access, semantic search, and feedback loops optimized for large-scale digital workforces
By co-locating these functions, AI factories eliminate latency between design, build, and deployment. They give companies more direct control over how their models are trained, where their data resides, and how resilient their infrastructure is to global shocks.
AI Factory Redefined
Nvidia now offers a fully integrated AI factory platform designed to accelerate this buildout. It includes not only hardware components, but a full software and orchestration stack. The TensorRT ecosystem supports high-performance AI inference, while Nvidia Dynamo and NIM microservices streamline deployment and model optimization. Data Flywheel enables continuous customization based on real-world feedback, ensuring that AI systems improve over time.
Nvidia’s full-stack platform allows AI factories to operate like industrial assembly lines for intelligence. Raw materials include foundation models, customer data, and toolkits. These inputs are shaped by high-performance training, fine-tuning, and inference, while continuous feedback loops allow systems to adapt and improve in real time.
The Nvidia Blackwell Ultra-based GB300 NVL72 solution provides 50x higher output for AI reasoning than previous generations. Nvidia’s DGX SuperPOD integrates this compute power into a turnkey system, while DGX Cloud delivers the same AI infrastructure virtually through major cloud providers. Both enable enterprises to scale rapidly without compromising performance.
To coordinate all these elements, Nvidia’s Omniverse Blueprint provides a method to simulate entire factories as digital twins, optimizing design, layout, and workflows in a virtual environment.
Transforming the Nature of Work
Unlike traditional manufacturing plants, AI factories don’t promise mass employment on the factory floor. Instead, they signal a shift toward high-skill, cross-disciplinary roles that include: 1) digital twin architects who model industrial workflows in simulation; 2) AI-operations engineers who monitor and optimize factory performance; 3) Specialists who program and maintain automated systems.
These jobs are fewer in number but higher in complexity and wage potential. In many ways, the AI factory reflects a broader shift from routine cognitive tasks to advanced cognitive skills. Such a shift demands the integration of expertise across computer science, statistics, manufacturing, and process management to solve problems holistically.
At GTC 2025 earlier this year, Jensen Huang unveiled “Blue,” a robot capable of learning tasks by modeling physical environments, a breakthrough underpinned by the same infrastructure AI factories now support. With mass production capabilities, AI factories will not only power digital models but fabricate the next generation of physical robots for logistics, manufacturing, healthcare, and domestic use.
AI factories thus serve a dual role: they support the software revolution while simultaneously enabling a AI hardware transformation.
The Environmental Challenge
But these benefits don’t come without cost. AI factories are energy-intensive and, if unmanaged, carbon-heavy. With Oracle planning a $40 billion investment in Nvidia chips for its Abilene, Texas-based Stargate facility, expected to deliver 1.2 gigawatts of compute, sustainability is a critical concern.
To mitigate this, Nvidia and its partners are implementing cutting-edge technologies. Liquid immersion and direct-to-chip cooling systems are being deployed to dramatically reduce energy spent on thermal management. Nvidia’s Blackwell GPUs, used in the GB200 NVL72 systems, deliver up to 25× more energy-efficient inference compared to previous generations, improving compute output per watt.
Meanwhile, some AI factories are co-locating with renewable energy sources to reduce fossil fuel dependence. Sweden, for instance, is supporting AI factories through green energy partnerships leveraging its abundant hydroelectric power. Microsoft’s datacenters in Finland have similarly emphasized low-carbon electricity sourcing.
Sustainability is now a design requirement, not a footnote. The future of AI factories may hinge not only on how much compute they deliver, but on how responsibly they deliver it, balancing energy efficiency with AI capabilities. As AI is moving from the cloud into an ecosystem of factories, the organizations and countries that take a lead in the long-term development of this terrain will shape the contours of global economy.