Packaging, Pricing And Selling AI
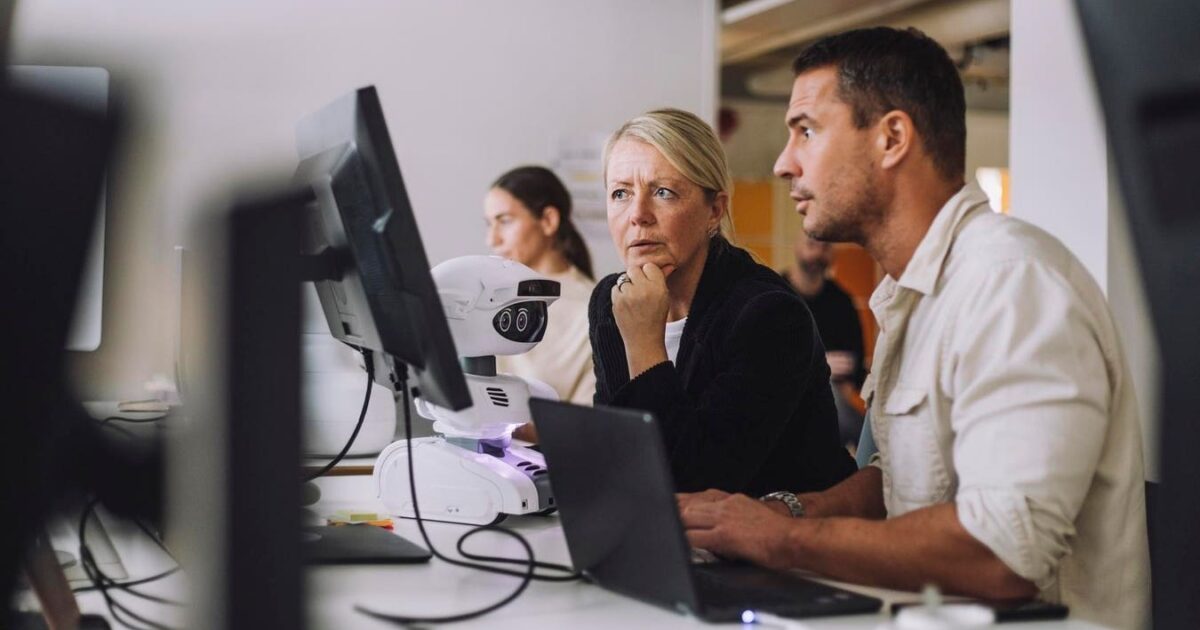
Su Belagodu is a seasoned Product Executive and a Start-Up Advisor.
The AI boom has ushered in unprecedented opportunities, but it has also created significant challenges in packaging, pricing and selling AI-driven products. As a product leader at an AI company, I have been deeply immersed in navigating these complexities firsthand. Through iterative learning and constant adaptation, we’ve uncovered key insights that are shaping our go-to-market (GTM) strategy.
Challenges In Packaging And Pricing AI Products
The Cost Dilemma
Unlike traditional SaaS, AI products incur substantial infrastructure costs, primarily due to expensive compute resources. The per-unit cost of delivering AI functionality, whether through API calls, inference costs or fine-tuned models, varies widely and can eat into margins if not accounted for properly. Many companies struggle to price AI products effectively because the cost structure is more fluid than typical software offerings.
Customer Skepticism And Explainability
AI-driven products often face skepticism around reliability and explainability. Potential customers want to know, “How much better is AI than my current solution?” and “Can I trust AI to make critical decisions?” Without clear packaging that communicates benefits, customers hesitate to adopt.
The Absence Of Established Pricing Patterns
As pricing AI is still an evolving landscape, traditional SaaS pricing models (seat-based, usage-based or tiered pricing) don’t always fit AI-driven products. AI tools may offer significant value even with low user engagement, making standard metrics like “per seat” or “per active user” insufficient. Furthermore, pricing AI solutions purely based on API usage or compute consumption can create unpredictability for customers.
Strategies For Effective AI Packaging And Pricing
Value-Based Pricing Over Cost-Plus Models
Given AI’s ability to drive efficiency, reduce operational costs and improve outcomes, pricing should be tied to measurable value. For example, an AI-driven automation solution can be priced based on cost savings in operational workflows rather than just charging per API call or per model inference. This resonates more with enterprise buyers who prioritize ROI.
Hybrid Pricing Models
A mix of fixed subscription fees and usage-based pricing allows for predictable revenue while accommodating variable costs. For AI automation tools, a structure that includes a base platform fee with additional charges based on usage (such as processing volume or model complexity) ensures customers feel they are paying for actual value while giving companies a cushion to manage AI-related costs.
Free Tiers And AI Explainability As A Selling Point
To combat customer skepticism, providing a limited free tier or transparent AI explainability reports can build trust. Offering AI-powered insights with detailed explanations of how the AI arrives at decisions reduces the “black box” effect that deters enterprise adoption.
Packaging AI As An Enhancement, Not A Replacement
Selling AI as a pure replacement for existing workflows can be a tough sell. Instead, positioning AI features as an enhancement augmenting human expertise rather than eliminating it helps drive adoption. Successful AI products are framed as productivity boosters that integrate seamlessly into existing workflows rather than as disruptive overhauls.
Successes And Learnings From The Field
Experimenting With Different GTM Strategies
Testing multiple approaches direct sales, product-led growth (PLG) and channel partnerships revealed that mid-market companies were more willing to experiment with AI-driven automation compared to large enterprises, which required extensive proof points. Research by Everest Group further backs this. For us, this led to an early focus on fast-moving digital-first companies that had immediate operational bottlenecks.
The Role Of Advisors And Customer Feedback
Engaging with advisors and gathering early customer feedback is invaluable. Pricing and packaging can be refined through continuous iteration based on what resonates most with customers. Advisor insights help recognize the importance of emphasizing cost savings and efficiency over purely technical differentiators.
AI-Augmented Sales Process
Leveraging AI internally for sales and customer support is also a game changer. Deploying AI-driven chatbots and knowledge assistants to handle initial customer inquiries can help sales teams to focus on high-value conversations. This not only streamlines the funnel but also provides a real-world showcase of AI’s capabilities.
Gradual Enterprise Adoption
While enterprises typically have longer sales cycles, demonstrating tangible AI-driven improvements and faster decision making can help build momentum. Positioning AI as an operational advantage—rather than a complex technological shift—was key to securing buy-in from IT leaders.
Conclusion
The AI market is still young, and best practices for pricing and packaging will continue to evolve. However, companies that remain agile by iterating on pricing models, incorporating customer feedback and tying AI costs to real business value can better position themselves to gain an edge in the market.
For AI product leaders, the key takeaway is clear: There are no fixed rules (yet), but a combination of value-driven pricing, hybrid models and transparent AI communication will be crucial in establishing market leadership.
Forbes Technology Council is an invitation-only community for world-class CIOs, CTOs and technology executives. Do I qualify?